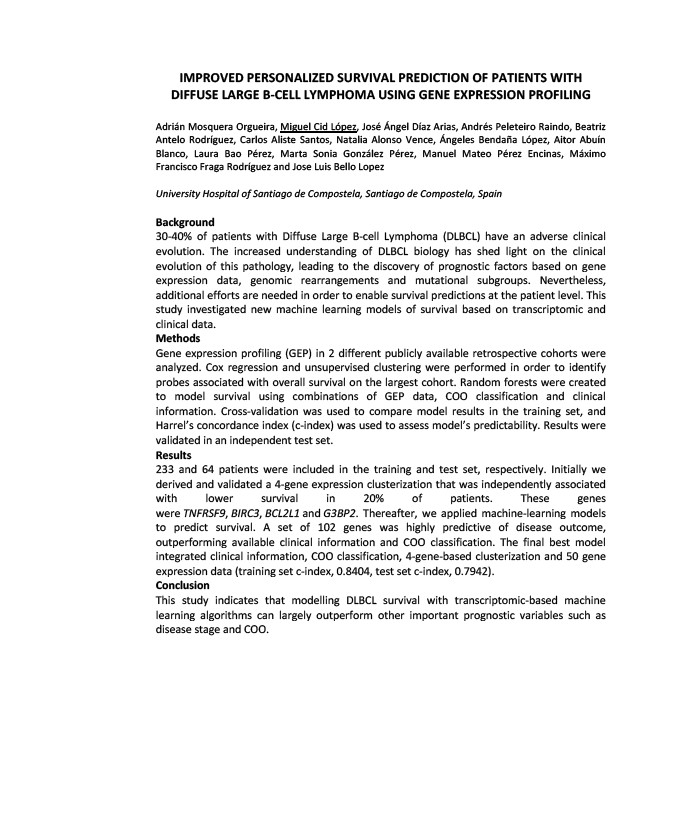
IMPROVED.PERSONALIZED.SURVIVAL.PREDICTION.OF.PATIENTS.WITH.
DIFFUSE.LARGE.B9CELL.LYMPHOMA.USING.GENE.EXPRESSION.PROFILING.
.
Adrián%Mosquera%Orgueira,%Miguel%Cid%López,%José%Ángel%Díaz%Arias,%Andrés%Peleteiro%Raindo,%Beatriz%
Antelo% Rodríguez,% Carlos% Aliste% Santos,% Natalia% Alonso% Vence,% Ángeles% Bendaña% López,% Aitor% Abuín%
Blanco,% Laura% Bao% Pérez,% Marta% Sonia% González% Pérez,% Manuel% Mateo% Pérez% Encinas,% Máximo%
Francisco%Fraga%Rodríguez%and%Jose%Luis%Bello%Lopez%%
%
University1Hospital1of1Santiago1de1Compostela,1Santiago1de1Compostela,1Spain1
%
Background%
30U40%% of% patients% with% Diffuse% Large% BUcell% Lymphoma% (DLBCL)% have% an% adverse% clinical%
evolution.% The% increased% understanding% of% DLBCL% biology% has% shed% light% on% the% clinical%
evolution% of% this% pathology,% leading% to% the% discovery% of% prognostic% factors% based% on% gene%
expression% data,% genomic% rearrangements% and% mutational% subgroups.% Nevertheless,%
additional%efforts%are%needed%in%order%to%enable%survival%predictions%at%the%patient%level.%This%
study% investigated% new% machine% learning% models% of% survival% based% on% transcriptomic% and%
clinical%data.%
Methods%
Gene%expression%profiling%(GEP)%in%2%different%publicly%available%retrospective%cohorts%were%
analyzed.% Cox% regression% and% unsupervised% clustering% were% performed% in% order% to% identify%
probes%associated%with%overall%survival%on%the%largest%cohort.%Random%forests%were%created%
to% model% survival% using% combinations% of% GEP% data,% COO% classification% and% clinical%
information.% CrossUvalidation% was% used% to% compare% model% results% in% the% training% set,% and%
Harrel’s%concordance%index%(cUindex)%was%used%to%assess%model’s%predictability.%Results%were%
validated%in%an%independent%test%set.%
Results%
233% and% 64% patients% were% included% in% the% training% and% test% set,% respectively.% Initially% we%
derived%and%validated%a%4Ugene%expression%clusterization%that%was%independently%associated%
with% lower% survival% in% 20%% of% patients.% These% genes%
were%TNFRSF9,%BIRC3,%BCL2L11and%G3BP2.% Thereafter,% we% applied% machineUlearning% models%
to% predict% survival.% A% set% of% 102% genes% was% highly% predictive% of% disease% outcome,%
outperforming% available% clinical% information% and% COO% classification.% The% final% best% model%
integrated%clinical%information,%COO%classification,%4UgeneUbased%clusterization%and%50%gene%
expression%data%(training%set%cUindex,%0.8404,%test%set%cUindex,%0.7942).%
Conclusion%
This% study% indicates% that% modelling% DLBCL% survival% with% transcriptomicUbased% machine%
learning% algorithms% can% largely% outperform% other% important% prognostic% variables% such% as%
disease%stage%and%COO.%
% %